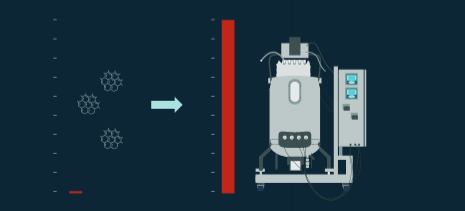
Intelligent Lentivirus Analysis – Coupling an Automated Imaging System with Artificial Intelligence
The number of gene and cell therapies in the clinic is increasing rapidly. With the increase in gene therapy manufacturing, comes the need for improved analytics during the production process. Analytics for Lentivirus production is particularly important as it is currently the second most common vector used in cell and gene therapy manufacturing (Figure 1).
Recently, Vironova presented a user-friendly method to improve characterization of lentivirus attributes during the manufacturing process. The method that incorporates artificial intelligence (AI) and their advanced imaging system is described in more detail in the attached infographic. We have summarized the highlights in this article.
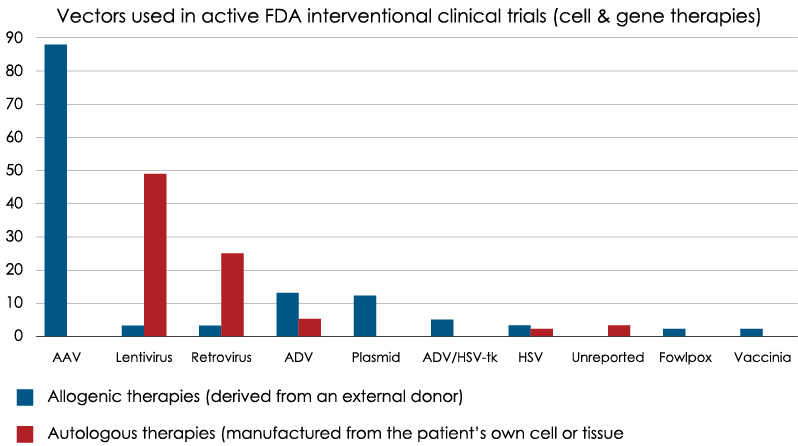
Upscaling Lentivirus – Production Challenges
Lentivirus is routinely produced at lab scale, however when larger quantities are required, scaling up from small to medium volumes can be quite difficult. Frequently the difficulty involves development of stable packaging cell lines. Another challenging area is finding efficient purification that maintains vector infectivity.
Common process failures:
Ideally, in upstream you have lentivirus with replication function that contains the therapeutic gene insert. However, you can instead produce a vesicle containing the therapeutic gene insert plasmid with no replication function or exosomes or vesicles partly containing lenti capside proteins with no therapeutic gene. In downstream, a purification process that isn’t optimized negatively affects the integrity or morphology of the virus. Analytics are necessary to accurately characterize and inform on the success of the manufacturing process and correlate process impact on critical quality attributes.
Particle Characterization Using Artificial Intelligence
Vironova has developed a method for particle characterization that couples their MiniTEM imaging system with AI, specifically convolutional neural networks (CNNs) to create a solution for characterizing lentivirus and other complex samples. CNNs are a form of AI inspired by biological processes in the animal visual cortex. CNNs require the use of classified data sets as trainers. In this case, classified data sets are created by identifying distinguishing characteristics in an image. By marking examples of what is of interest in the images, the neural network on the computer learns to characterize these images. Results have been demonstrated to be similar to what you would expect when an image expert does the analysis.
The way that Vironova explains the technology is that the AI in your phone learns better face recognition with every picture. Similarly, the MiniTEM learns virus sample analysis by training with a large image database of viral samples. As a result of this training, the system now requires minimal interactive training and fine tuning to work for various applications.
Vironova points out that there are pros and cons to using AI for image analysis. On the positive side, you can achieve better results, remove the need for an image expert to design each step of the analysis, and it is more user-friendly. The negatives are that AI requires lots of training data, only learns what it sees, and requires expertise for suitable design of the network architecture.
Case Study – Lenti Analysis
In the infographic, Vironova shares a case study where their objective was to evaluate a filter step in a downstream process for lentivirus production.
To conduct this work, they used MiniTEM imaging coupled with AI (Figure 2). After training the MiniTEM, Vironova was able to effectively identify Lenti particles from other debris.
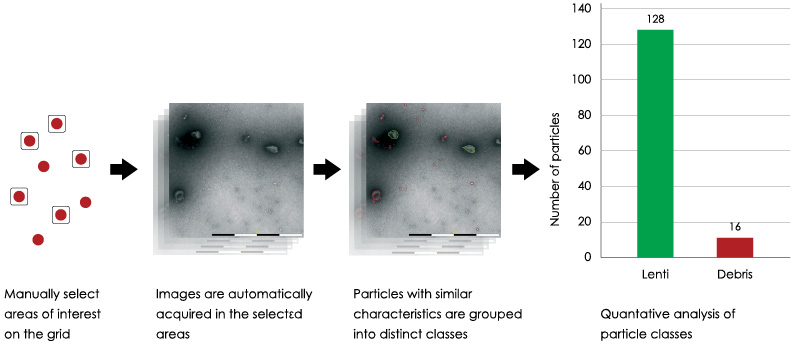
Vironova then demonstrates how indirect methods, like ELISA, can be highly inaccurate (Figure 3). In one of the samples from the study, using ELISA, a high concentration of P24 was detected, but when looked at with the MiniTEM, it was clear that there were actually no intact Lenti particles present.
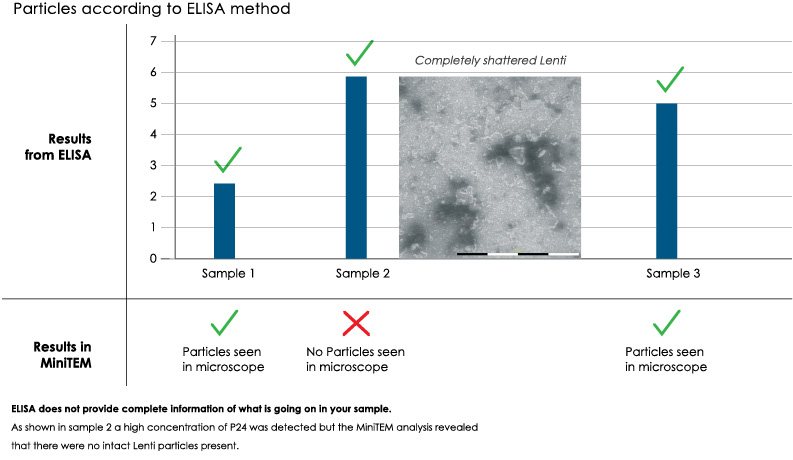
Next, they used the MiniTEM to look at the Lenti sample after clarification and again after filtration to evaluate the impact of the filter step (Figure 4). For this specific lenti analysis, Vironova found that the downstream purification steps affected the integrity of the lenti particles in some cases. They were able to enrich the lenti particles, but were not able to purify out the smaller vesicles.
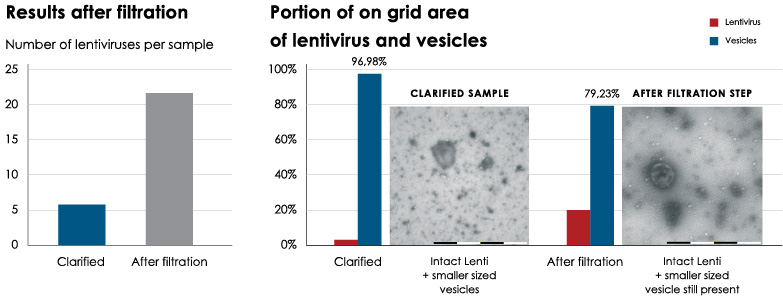
Summary
By using automated imaging via the MiniTEM and AI, Vironova created a powerful tool for characterizing complex samples such as lentivirus. The analysis provided valuable information about the effect of different purification steps and could be used in a similar way to study the impact of other process steps as well.