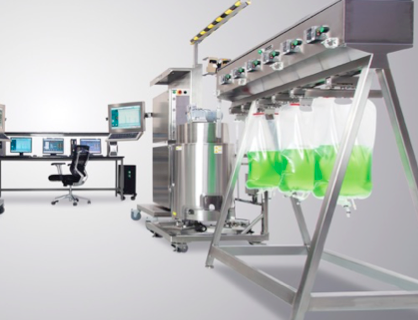
Is Automation the Disruption Pharma R&D Needs?
A Guest Blog by Barbara Paldus, PhD, CEO and co-founder, Finesse Solutions
According to a recent report from PhRMA, U.S. biopharmaceutical companies spend more than 13 times the amount of R&D per employee than all other manufacturing industries.(1) This is because potential drug candidates require lengthy and complex testing to ensure they are safe and effective for patients. Yet, despite all of the money invested, only about 12 percent of the drug candidates that make it into Phase 1 testing are approved by the FDA. While knowledge about a disease and its potential treatment is not lost with “failed” candidates, innovative research tools must be used to avoid spending time, money, and effort on products that have limited endpoint efficacy. Through the implementation of automation, data management, and analytical technologies, pharma has the opportunity to breathe new life into a number of areas of the biologics drug development process.
Optimizing Pharma for Efficiency and Accuracy
Technology and automation have completely transformed our world, from how we communicate to how we conduct business – and even how we spend our leisure time. They have had a profound impact on almost every industry, but most notably on industries such as finance, automotive, chemical, and home management. In manufacturing, it has become clear over many decades that technology and automation can be used to drive quality and efficiency. These benefits are especially critical in the pharmaceutical industry, where there is an added focus from companies and regulators on the use of technology and automation to improve drug development processes. For example, the FDA process analytical technology (PAT) initiative focuses on improving the understanding of manufacturing processes using data analysis, process monitoring, and continuous feedback. Through the continued development and application of automation, non- value-added activities are eliminated, allowing for increased speed, efficiency, and accuracy. The same potential rings true for technology and automation in drug development R&D, where pharmaceutical scientists and researchers are bogged down with manual tasks such as bioreactor cleaning and tubing management, or manual data entry and retrieval. In addition, because the systems used to run experiments are fairly complex, the people who design the equipment are often the only ones who truly know how to run them. This is commonplace in smaller companies where there are fewer resources. Through the use of automation, R&D experts can alleviate the burden of many of the aforementioned time- and labor- intensive tasks. Their time can then be used to focus on activities that leverage their expertise and extensive training, such as guring out the metabolic pathways to optimize cell lines and expression systems and selecting the optimal way to increase titer. Appropriately designed user interfaces for automation systems can also enable scientists to create sophisticated design of experiments (DoE) to collect and analyze valuable data.
Furthermore, R&D experts and their clinical counterparts can perhaps avoid facing the difficult and time-consuming task of determining how to scale up a process that was created with very simple lab equipment to one that is run in a sophisticated and automated manufacturing environment. In the event of regulatory approval, having the R&D and cGMP processes automated with the same platform facilitates rapid tech transfer and saves on both time and associated cost of scale-up/scale-down.
Using Data to Make Better Decisions
In today’s research environments, data is often collected manually and stored in Excel spreadsheets. Not only is this time-consuming and prone to error, but the data is often not collected in real time. While spreadsheets are useful, they are not the optimal database for this field. Databases (such as SQL) for the upstream and the downstream process data can be used to form a library from which global process optimization can be achieved. Additionally, having this data and real-time analysis provides researchers (or the manufacturing group) with the capability to detect issues as they arise. This allows some ability to proactively implement change as needed and eliminates the time spent collecting batch statistics to determine where errors may have occurred. By leveraging technology and smart automation systems, knowledge about multiple areas of the drug development process is gathered into one interface, where scientists can analyze it and make connections between observations.
Finally, as regulatory oversight moves closer to R&D, the integration of analytical information from R&D process runs, data management, and data harmonization become critical. For development and scale-up of a process, accurate data management and accurate process measurement are needed from the beginning of when the cell line was developed. This batch data can then accelerate the regulatory review by providing additional support to demonstrate batch optimization and reproducibility, which is often a high-risk, high-dollar part of the regulatory approval process. In the future, as process analytics become an integral part of the batch record and regulatory approval review, the data about the process will become as important as the process itself; only then will the FDA PAT initiative, defined in the Guidance for Industry PAT as a “Framework for Innovative Pharmaceutical Development, Manufacturing, and Quality Assurance” truly fulfill its original promise.
Doing More with Less: The R&D Budget Struggle
A 2016 global life sciences report from Deloitte identifies squeezing profit margins, patent expiries, and rising R&D costs as factors driving more focus on operational efficiencies.(2) Taking into account the increased scrutiny of healthcare costs, the explosion of the biosimilar market, and global competition, it becomes clear that pharmaceutical companies are faced with a daunting task of delivering innovative therapies while maintaining profit levels and maximizing shareholder value. The report notes, “Achieving these often-conflicting objectives is likely to require that companies transform their business and operating models and embrace disruptive technology advancements that can concurrently reduce costs and speed time to market for new products and services.” While the majority of new drug development costs are still embedded in the clinical trials, having a robust and scalable production process for the drug is equally critical so that revenue can be quickly generated post approval. Therefore, efficient process development remains crucial to commercial success.
An example of this type of process transformation is embodied in the Finesse® SmartLab™ platform announced earlier this summer. SmartLab includes the Finesse TruBio®, TruPur™, and TruChrom™ bioreactor control software suites and leverages the G3Lab™ Universal hardware platform that is being rapidly adopted by the R&D community. In addition, the SmartLab system allows for the harmonization of analyzers and bioreactor controllers from a wide variety of suppliers while also offering statistical analysis tools and electronic batch reporting. This flexibility enables researchers to move away from using Excel spreadsheets and gain efficiency in the development of new biologics. This in turn reduces the R&D costs of investigating new drug candidates that are still at high risk of failure.
SmartLab is the first end-to-end data management system to integrate leading third-party DoE software, multivariable data analysis (MVDA), recipe management, data visualization, and seamless analyzer connectivity. This offers life sciences research and process development groups a novel and cost- effective data archiving solution, centralized data/ recipe mining for DoE or statistical analysis, and remote access to process reports, plots, and live trend visualization against a “golden batch.” SmartLab can also notify researchers by email or text when batch reports are ready for viewing, when online values are out of range, and when network connections are down.
The Final Step: Scaling from R&D to cGMP
To satisfy the needs of late-stage regulatory testing (Phase 2 and 3), the R&D process must be scaled to batch sizes that are at least several hundred liters. This intermediate step between research and full production also serves as an opportunity to optimize cell productivity and viability. However, in today’s facilities, the research automation tools are often provided by different vendors than the larger-scale development and production tools. This leads to a difficult transition due to a lack of cohesive data management and confusion around process parameter transfer.
An effective approach that avoids many of the aforementioned pitfalls was announced earlier this summer. Iceland biopharmaceutical company Alvotech opened a hybrid facility in the University of Iceland Science Park that leverages automation in an effort to drive operational efficiency.(3) According to the release, the facility enables the company to “produce higher yields, significantly reduce labor and capital expenses, and maintain flexibility in operations management systems.” It uses the same automation in both R&D and production to enable rapid scale-up and technology transfer using the Finesse TruBio, TruPur, and TruChrom platforms. Through the use of these systems, a process developed in a 10-liter glass vessel can now be rapidly scaled up to a 50- or even 1,000-liter scale in process development and then transferred as an electronic recipe le to a 2,000-liter production scale. Other advantages of this approach to global factory automation were outlined in the announcement:
The open architecture allows Alvotech to choose the equipment best suited and priced for its operations, regardless of equipment manufacturer in both R&D and cGMP production.
Process flow can be designed in a modular and scalable manner while maintaining quality and regulatory compliance in electronic batch records at the same time.
The flexibility of the system lets a user select the level of automation required for each unit operation in both R&D and cGMP production.
Users can focus simultaneously on the compatibility of single-use materials between upstream and downstream for global process yield optimization. The same materials are used from R&D through cGMP, just at different volumetric scales.
Overall, these types of smart facilities demonstrate “the ability to quickly move from upstream to downstream automation without compromising the project budget or timeline.” It is this type of change that will allow the industry to survive in a new era that requires a fine balance among innovation, efficiency, and cost.
References
[1] PhRMA, 2015 Profile, Biopharmaceutical Research Industry
[2] Deloitte, 2016 Global life sciences outlook: Moving forward with cautious optimism
[3] BioProcess Online, Finesse Smart Factory Platform Featured New Manufacturing and Lab Facility in Iceland