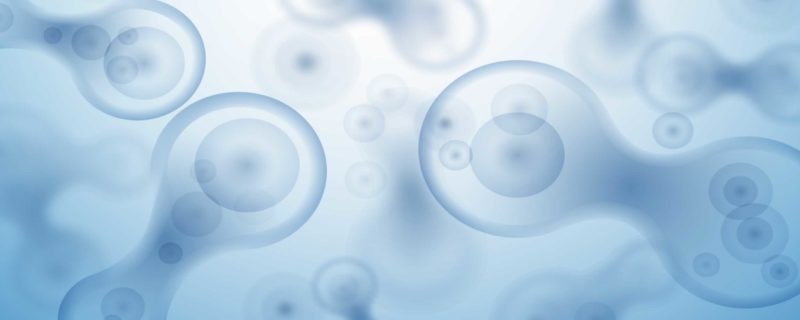
Out with the old, in with the new: cleaning-up your methods
It’s not surprising that many scientists are creatures of habit. After all, the “re” in “research” often feels like it stands for “repeatedly”. This attitude means that more often than not, scientists will develop a preferred “go-to” protocol. These are the ones that have been run countless times over the years, and become the protocol to learn for anyone new to the group.
However, this proclivity towards the familiar can unwittingly cause issues, especially in today’s rapidly changing scientific and technological landscape. The tried-and-true protocols of the past may fail to achieve their full potentials without constant introspection and periodic maintenance/updating.
Here, we’ll look at a few key areas where that old, faithful protocol could use some adjustments, and offer some suggestions on how to get the most out of your methods.
Back to basics: reproducibility, reliability, refinement
Every scientist at some point or another has likely asked “how can I optimize my protocol?” Well, the first step is to carefully examine it and understand the purpose of each step. Second, you have to carefully check that your protocol generates consistent, reproducible, accurate, and precise results. Finally, you have to refine and optimize your protocol for efficiency, so that you’re getting the most out of every sample and every second.
Starting with reproducibility, the key to ensuring that each run is the same is minimizing as many variables as possible. Where can variables be introduced? Some result from interoperator differences, some are from equipment calibration inconsistencies, and some come from using different batches of reagents. It’s important to make sure that all the operators are on the same page, using the same reagents, the same equipment, and the same methods.
Instrumentation plays the biggest role in ensuring reliability, especially in this day and age of software data analysis. Your data is only as good as the sensors, detectors, and algorithms used to generate it, and it’s up to you to regularly examine all three aspects. Don’t forget about the little guys either. Newer instruments have detailed calibration metrics and protocols included in the manual and on-board software, but your old-fashioned balances and pipettes may still be the backbone of the lab, and they need regular inspection as well.
Finally, we come to refinement. Every second is precious: look for workflow inefficiencies. How long does the protocol take from start to finish? Can it be completed within the average working day? Are there awkward timings requiring someone to stay late or come in for the exclusive purpose of performing a single step? Can you start the protocol at 4:30 PM, or do you have to wait until the next morning because of logistics? Remember – your irreplaceable quality as a scientist is the ability to intuit, to reason, and to think. Don’t waste your days pipetting when there’s a system to do that for you.
Reproducibility is in your hands: good laboratory practice
Interoperator variation can creep into a protocol in many ways. For example, over time, experienced operators may have tweaked and adjusted protocols without documenting these changes, leading to different results when a new user takes the helm. While these tweaks are mainly prevalent in non-automated workflows, concerning so-called “caretaker” steps such as washes, they can extend to semi-automated and automated workflows in the guise of software settings and analysis thresholding parameters. Don’t neglect knowledge as a resource either. It’s no secret that laboratories have high turnover, meaning that the expert who originally devised and rigorously tested the protocol is potentially no longer with the group. Proper documentation of both the original protocol and any subsequent adjustments is absolutely critical, both for the success of the assay and for regulatory purposes. Don’t just let precious information disappear out the door.
Another opening for variability lies with the reagents. Not all reagent lots are the same, especially if the reagent supplier has changed over time. Furthermore, are different operators drawing upon their own private reagent stashes? Depending on how often said individuals operate the protocol in question, these stashes can greatly range in age and storage conditions. If the laboratory shares a common batch, who is responsible for making and maintaining the stock? In non-automated and semi-automated workflows, does everyone use the same instrumentation and perform the protocol in similar environments? For example, different operators may use different incubators for their cells. Does this affect the distance that the cells travel from the incubator to the liquid handling apparatus?
A lot of these issues seem very minor on the surface, but small, insignificant discrepancies multiply, and can exert noticeable effects on the final result, especially if they’re at the start of a workflow, and operator vigilance is required. At the same time, many of these issues can be rectified through automation. The utilization of a fully automated liquid handling apparatus, such as the Beckman Coulter Biomek Automated Workstations, can help maintain reagent and instrumentation consistency across operators. It can also enforce protocol uniformity, given that any adjusted methods still have to be programmed into the system (and likely saved for future use) before they can be executed. This, if diligently maintained and monitored, helps prevents knowledge degradation, as the protocols of previous users can still be stored in the system and referenced.
Everyone needs a checkup: instrument reliability
Another key component to limiting experimental variability is making sure all instrumentation and equipment is operating properly. It can become habitual, especially for more complex instruments, to simply add the sample, hit a button, and wait for the results. But many interactions and processes went into the generation of those results, and it’s important to be able to trust all of them. Make time for routine maintenance so that small miscalibrations don’t turn into catastrophic failures.
Over time, all instrumentation accumulates wear-and-tear. There is a tendency to allocate maintenance time and resources based on instrument cost. But don’t forget the little guys. Balances, hot plates, water baths, thermometers, and the like aren’t the flashiest things in the laboratory, but their accuracy is absolutely critical to every experiment performed in that room. Manually-operated equipment, such as pipettors and pH probes, can be especially suspect. One slip of the hand and that delicate tip or probe might hit a glass wall or a benchtop. One jerky thumb motion and fluid could be aspirated into the vacuum chamber. These instruments require the most attention to ensure interexperimental consistency.
For larger, more complex instruments, wear-and-tear can be more subtle. Failing sensors result in altered detection thresholds, but the difference may be so slight from run to run that the operator thinks nothing of it because it falls within normal sample variation. But a 0.1% difference each run becomes a 10% difference after 100 runs, and when you finally realize it, how much data do you exclude? Or do you redo the entire 100 runs? Alternatively, the researcher may have moved on to a different experiment altogether, and without a suitable comparison, mistakenly interpreted this skewed data as the appropriate baseline.
Instrument maintenance is particularly important higher up the workflow. Equipment used during standardization assays, such as cell counters to check well density, spectrophotometers for protein level quantification, and of course, liquid handling tools at all times, need to be precise. Any errors at this point will snowball and invariably invalidate the entire protocol, and worse, this may not be detected by the operator. It is very difficult to distinguish uniformly skewed calibration data as erroneous, as your standard curve samples are subject to the same skew.
For manual or semi-automated workflows, calibrating and maintaining multiple physically disparate pieces of equipment can be time- and labor-consuming. The adoption of a fully automated workflow can alleviate this problem, not only by physically clustering all the relevant instrumentation within a centralized workstation, but by automating and integrating diagnostic procedures and providing technical support.
Remember, equipment issues are generally harder to detect. The usual benchmark for protocol function is reproducibility. Operator errors induce variable skew – one experiment is high, the next is low – but instrument errors induce constant skew – all experiments are off in the same direction. Don’t wait for something to go wrong. Perform routine, and ideally automated, maintenance using standards or benchmark values established by a separate instrument.
Refining your protocol: the simplicity of automation
One thing about protocols is that they rarely neatly align with your daily schedule. This tends to lead to either more stress for the scientist when they have to perform a simple caretaker step at 11:45 PM, or it leads to variability when the definition of “overnight” can vary by hours. Clearly, neither of these is an optimal outcome.
Manual workflows take significant amounts of time and energy, and this decreases research efficiency. Time spent pipetting could be better dedicated to more productive things like reading, planning, interpreting, and thinking – all things that scientists are uniquely qualified to do. The energy expended through not only physical movements and manipulations, but also the high levels of concentration required, leads to mistakes, the need for rest, and even injury. To optimize protocol efficiency, let machines perform mechanical actions. A fully automated system is not only capable of higher throughputs, it can also be pre-set to run during evenings, lunch breaks, and weekends. If automated liquid handling systems are integrated with other instruments (e.g. incubators, cell counters) as part of a workstation, they are capable of performing multi-day protocols requiring minimal human observation/intervention.
Another way to improve efficiency is to look at data collection and analysis. The success of any protocol does not only rest upon the ability of the user and equipment to perform it properly, it also lies in the ability of the user and equipment to determine whether it was performed correctly or not. Manual data collection is again labor-intensive, and can be subject to observer bias, sample size restrictions, and sampling inconsistencies. The use of automated analysis software can greatly ease this burden, but also needs to be routinely examined. Is your software user-friendly enough to allow you to understand where all those numbers on the screen are coming from? Are you using a default software template generated a decade ago without reviewing the settings and thresholds? All of these things reduce the accuracy of your protocol, and could significantly influence your ultimate conclusions.
The importance of teamwork
Ultimately, every aspect of a protocol, from the operator, to the reagents, to the instrumentation are a part of a team. A good team relies on each member playing up to their strengths and letting other team members compensate for their weaknesses – to just do their jobs. Employ the strengths of automation so that you can enjoy better productivity and efficiency, while employing your strengths and taking your research to the next level.
For more information and tips about spring cleaning your methods please see – http://info.beckmancoulter.com/newmethods