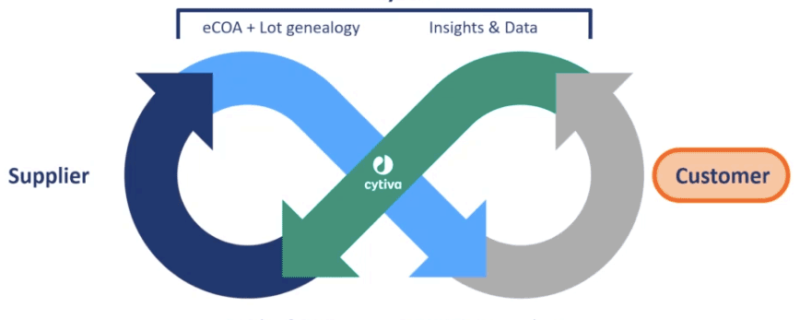
Reducing Raw Material Variability and Increasing Cell Culture Process Control
Over the past decade, the biomanufacturing industry has developed a greater understanding about how variability in raw materials can impact manufacturing processes and final product quality. This understanding has been possible primarily due to new analytical technologies. These new technologies have provided better tools for collecting, analyzing and reporting on raw material data sets that are key to characterizing raw materials. This has in turn led to a better understanding of the manufacturing process and has led to a more defined and optimized process.
When raw material variability issues arise, the cause must be determined and manufacturers and suppliers must work together to make necessary changes to minimize variability. Variability can be reduced across the biopharmaceutical value chain by characterizing raw materials, monitoring materials quality, and controlling raw material usage.
I recently viewed a webinar that provided a detailed example of how cell culture process variability can be controlled using supplier enabled data management and analytics. During the presentation, Aaron Woolstenhulme, Digital Commercial Leader, Cytiva, described how Cytiva’s cell culture manufacturing site in Logan, Utah is implementing digital biomanufacturing to better control and analyze raw material to reduce variability.
Webinar Highlights
Mr. Woolstenhulme began by sharing Cytiva’s motivations for the move to digital biomanufacturing. The first motivation was to get connected by taking in data from their raw material suppliers, defining global specifications for raw materials and connecting lab and production equipment. The second motivation was to gain insight by gathering analytics on both material and process and through data collaborations with end users, find out if Cytiva products are working as expected and what can be done to improve performance. Lastly they want to use this information to optimize their production and implement controls to eliminate, mitigate or minimize raw material variation.
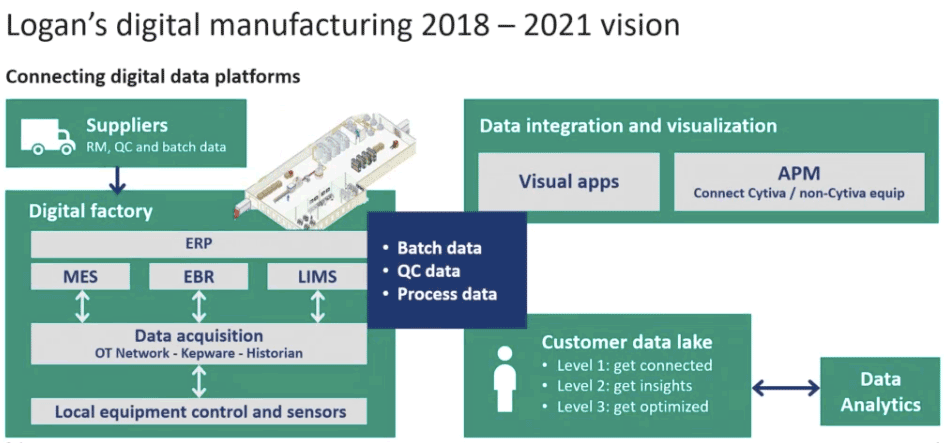
Aaron explained the digital data flow that Cytiva is aspiring to create, where data flows across the supply chain from supplier to Cytiva to customers and back again, enabling insights to be shared and improvements to be made across the supply chain (Figure 2).
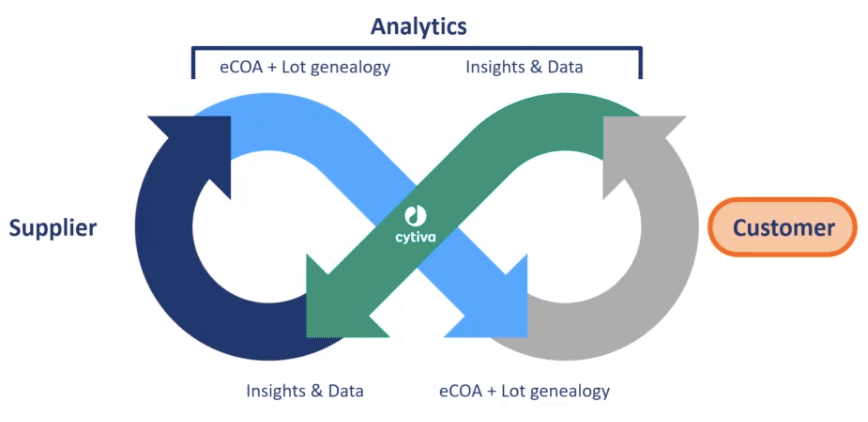
Using Digital Biomanufacturing to Improve Cell Culture
Aaron then explained how the digital transformation would inform and improve cell culture products. Characterizing raw materials uncovers the source of variation, allows detection of variations and informs about the impact that these variations have on the process and ultimately product attributes. Variations can be detrimental or beneficial to cell culture performance and the data collected will help Cytiva make sure that their customers are getting the right materials based on specific cell line needs. Increasing supply chain visibility also enables a risk-based approach to raw material variation.
Risk-based Approach to Raw Material Characterization
A key part of Cytiva’s approach is to use a risk-based approach for raw material characterization. Aaron explains that because thousands of raw materials are sourced for use in cell culture media, feeds and other process liquids and buffers a comprehensive characterizaiton of all raw materials is not possible. Thus, a risk-based prioritization of raw material characterization is required. While all raw materials have baseline requirements and specifications, high risk materials should be further characterized. High risk materials are identified using a set of defined rationale and trace metals has been identified as an area for further study.
Case Study – Impurity Analysis of an Iron Carrier
To demonstrate their risk based approach, Aaron shared a case study in which an iron carrier was examined (Figure 3). Iron can be an ingredient in cell culture media, but it is often the cause of variation in media performance. For the case study, they looked at 14 lots of iron carrier from 7 different suppliers. Samples were prepared of equivalent masses and 70 different elements were analyzed using ICP-MS. They found multiple other elements in the iron. He said that based on the data, the easy answer looks to be use pure iron, but that wasn’t always the case. They found through their data colaboration with customers that there were mixed performance results using high purity ingredients. It was found that some of the cell lines needed a level of impurity to perform as well as expected. Of course this was cell line and media formulation dependent. However, the further analysis allowed Cytiva to see which cell lines were sensitive to specific impurities and thus make appropriate adjustments.
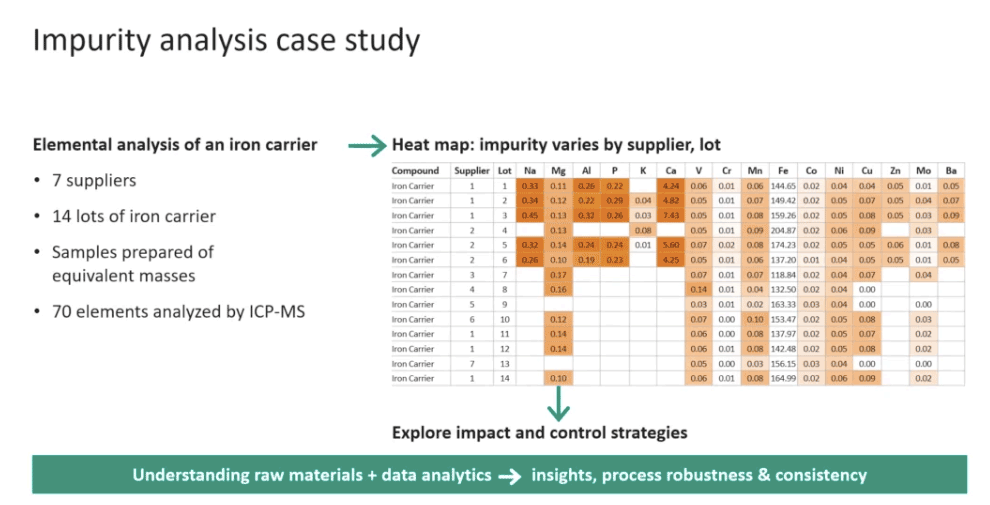
Predictive Modeling and Targeted Manufacturing
When Cytiva discovers a specific cell line sensitivity, they use data to select the appropriate combination of raw materials. First they create a data lake based on feedback from customers and then run an analytical script using the aggregated data. For example, in order to not exceed x parts per million of copper as an impurity, they use analytics to identify which material from which lots should be used to achieve the desired specification (Figure 4). After the script has run, the finished good is then tested to ensure it meets specifications. Having this data allows Cytiva to pick the best combination of raw materials for a more targeted manufacturing of cell culture media.
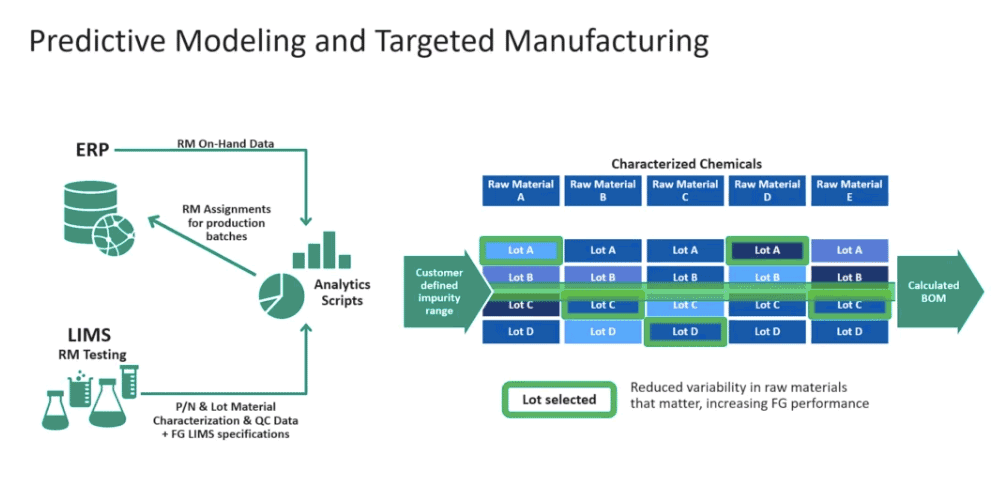
eData is Needed for Efficient Communication
In order to really make digital manufacturing work and the infinity digital data flow, eData is key. Cytiva provides a Certificate of Analysis (eCOA) and lot genealogy data in one electronic file that follows current ASTM XML standard formatting. With eData, it is possible to send specific product information before customers even receive the material.
Conclusions
Collaboration between raw material vendors, suppliers and manufacturers can result in improved quality and reduced variation. Aaron said that we still have some way to go and it will take a commitment to digital data sharing on raw materials and processes, however the resulting transparency will empower both suppliers and customers to take more control of raw material variability based on data analytics.
For more information, please see the webinar, “Controlling cell culture process variability through supplier enabled data management and analytics.”