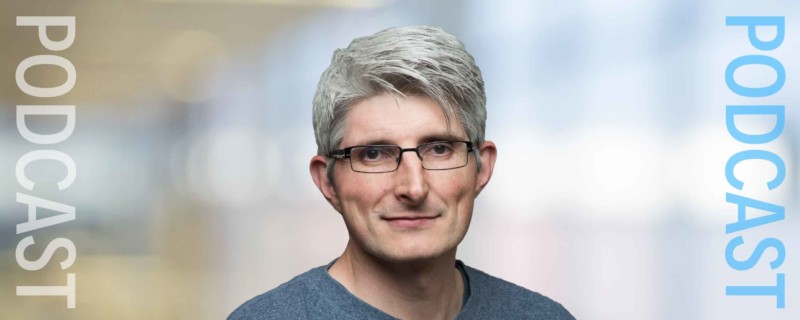
Computer Aided Biology Platform Helps Companies Meet the Challenges of 21st Century Biomanufacturing
Podcast: Download (Duration: 26:17 — 36.1MB)
Subscribe Here: Apple Podcasts | Spotify | RSS | More
Subscribe to the Cell Culture Dish Podcast on: iTunes | Google Play
Show Notes:
I began the interview by asking Mr. Gershater to describe the concept of Bioprocessing 4.0 and what it means to the industry. Markus explained that the term 4.0 refers to the industrial revolutions that have happened throughout history. The first began when steam was introduced as a power source, next came electrification and the production line. 3.0 refers to the incorporation of automation and 4.0 is the connection of different devices and automation through digital technology. This involves cloud computing that enables data storage, computing and analysis. This is particularly important in a complex industry like bioprocessing that requires sophisticated knowledge and control. Bioprocessing 4.0 will enable the industry to progress to the next level.
Next I asked Markus to explain the solutions that Synthace provides in this area. He described how Synthace started as a bioprocessing company that was looking for a way to conduct more sophisticated, automated experiments. The result was the creation of their software Antha, which can auto generate instructions for biological protocols. This means that scientists can specify the protocol they want to run and Antha will works out all the details down to the every step of the run. It then converts those detailed actions into scripts for each automated device to run the protocol. The user hits go and the robot will run the specified protocol with the instructions that Antha generated. This automatic generation of scripts makes automation more user friendly and powerful. In particular, there is a problem with lab automation due to the complexity of programing it. Antha is able to make complex lab automation implementable.
Markus goes on to say that the beneficial knock on effect is digital integration. The devices used in protocol automation are only a small part; there are also analytical devices that produce data. What is needed is a way of structuring data from all of these diverse pieces of equipment. Since Antha generates all the detailed instructions that go on into a particular protocol, it also has the detailed structure of the experiments. So at the end of any chain of actions, Antha can provide the provenance of all data points. Thus, it can also auto structure data into the context of experimental design.
As the industry runs more complex and high throughput experiments, the bottleneck shifts to data structuring. Antha has become a tool that allows the automation of lab processes as well as the data processing from those lab processes. This permits dynamic updating as the structure of the experiment updates.
We then discussed the technology behind the product. Markus explained that first step in getting started is to identify a specific protocol. Then, for example, Antha specifies samples that need to be diluted and provides a framework with specific parameters. Next, you need to look conceptually at how you can move liquids around to fulfill this design. What equipment do you need to run and what consumables? Once you have those, Antha can generate the lower level tedious details. This allows users to change one detail of the experiment and Antha will calculate a new set of instructions. Antha can then pass these specific instructions to devices through the Antha hub, which communicates with the equipment. Once users are satisfied that the equipment has been set up properly then they can hit go and the experiment will run.
I asked if there were any case studies that could be shared to show how this would work in a real life setting. He described how their case studies range from programming relatively simple workflows like automating ELISA assays to extremely complex experiments. They recently co-published a study with Biomedica where they ran an experiment to improve their process for generating lenti viral vectors and were able to improve viral vector titer ten fold over the course of just two very sophisticated Antha experiments.
Markus shared that Biomedica is good at automation and programming automation. When they looked at the scripts generated by Antha, they determined that it would have taken them a week to program each experiment that Antha generated on the fly. He says that this illustrates why often automation isn’t used. Scientists don’t have time to spend a week programming automation for an experiment that they might only run once. There is not sufficient return on investment for the time it took to program.
Synthace has also generated case studies around automated data structuring. In this example, he explained that with bioprocessing you have bioreactors and sensor data that must be aligned with sample data to provide a full picture. Antha enables this data structuring.
Next, I asked Markus if he could talk a bit about the vision for computer-aided biology and how he sees the evolution of the space in the next five years. He explained that computer-aided biology is a vision of how we can use twenty-first-century tools to help us pick up on these complexities of biology. This application can give us insights that maybe wouldn’t have been possible without applying machine learning. This doesn’t mean replacing scientists and engineers with AI, but instead flagging things that they may have missed due to the highly complex data sets.
He said that at conferences, there has been a growing swell of excitement around using these methods for drug discovery. However these techniques are just as important in the lab to interpret bioprocessing results. To reach this sort of future, that includes AI augmented insight, requires routine production of highly structured data sets every time and with every experiment, so that you can compare results experiment to experiment.
Frequently there is an expectation that scientists and engineers should be conducting the data structuring, but it is highly onerous. There is also a wide range of techniques being used to do this from company to company. There needs to be a system in place, where as much automation is incorporated as possible. This will open up the opportunity for an ecosystem of hardware and software working together.
This led me to ask the next question on building a common culture between science and software. Markus explained that this it is interesting because scientists and software engineers tend to think of things in fundamentally different ways. Biologists are used to a large amount of ambiguity because they deal with such complex systems on a day-to-day basis. For software engineers on the other hand, things are a lot more defined and a lot more predictable. They are used to making things happen in a powerful way very quickly.
He said that it is fun to see them work together to discover what is possible and what’s not possible and learn from each other. He goes on to say that what is nice about the Antha system is that both sides can understand it – scientists want to use it to automate the protocol and software engineers can see the logic within the protocol because it is highly defined.
He then told a story about hearing a speaker from Amgen discuss this same point and she said the common culture is “just happening naturally” as more digital tools are available and scientists are shifting their mindset about how to conduct their science.
To learn more about Synthace and computer-aided biology, please see…