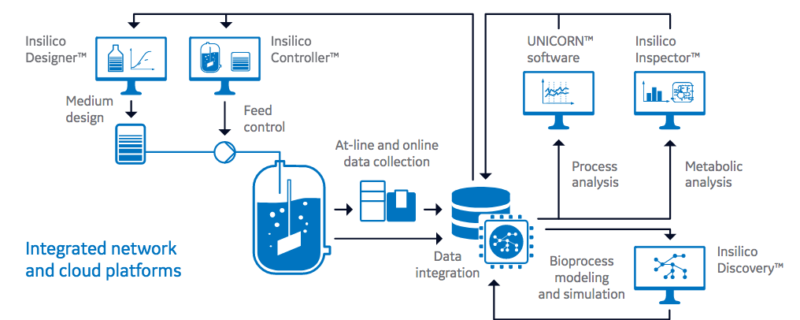
Implementing Digital Biomanufacturing in Process Development
At this year’s Bioprocess International Conference in Boston, Dr. Thomas Seewoester, Amgen gave a very insightful and inspiring keynote presentation titled, “Bioprocessing 4.0 – Digital technologies are transforming biologics manufacturing.” In the talk, he discussed the evolution of biomanufacturing and how digital technologies were a large part of the next step forward. He reminded us that each company is moving at its own pace and that the level of digital implementation is varied across the industry. He encouraged us as an industry to think outside the box and to look at how other industries are incorporating digital technologies to see what we can apply to biomanufacturing.
Digital biomanufacturing is an important step forward and certain areas within that process could benefit greatly from implementation. For instance, process development has been both a critical and resource intensive part of biomanufacturing. To fully maximize process development also requires the collection and management of large amounts of data from various sources, thus it stands to benefit greatly from the aid of digital technologies. A recent article titled, “Process development in the digital biomanufacturing age,” addressed just this issue. The article was published in the “HTPD – High Throughput Process Development,” a group of expanded abstracts covering talks presented at the fourth High Throughput Process Development Conference in Toledo, Spain. The article highlights the use of digital manufacturing concepts to improve predictive modeling and speed process development workflows.
Authors, William Whitford, GE Healthcare Life Sciences and Daniel Horbelt, Insilico Biotechnology, discuss the ways in which digital technologies are impacting biomanufacturing and specifically process development.
Authors point out that we are already starting to see the benefits of incorporating a digital biomanufacturing approach in areas including:
- Process understanding, monitoring and analytics
- Plant design and automation
- Process design and flow
- Embedded, distributed, or modular control units
- Enterprise resource planning and manufacturing execution systems
Key initiatives in the continued advancement of digital biomanufacturing in process development include de-siloing data, predictive simulations, model reference adaptive control, dynamic enterprise control algorithms and process automation.
Authors explain that predictive modeling is an integral part of unlocking the full potential of digital biomanufacturing. Accessing a wide variety of data including bioprocess history records, at-line analytics and research databases and coupling that with well-designed algorithms provides both “quantitative understanding of cell physiology and advanced model-based control toward process development, operational efficiency, and business goals”.
Another important key is creating intelligent software to collect and process large amounts of data from a variety of sources. The authors point out that intelligent software applications can analyze bioprocess data along with metabolics, transcriptomics or proteomics data to generate individualized metabolic network models to represent specific host cell lines and bioprocesses. Figure 1 provides an overview of intelligent software applications in digitalized process development.
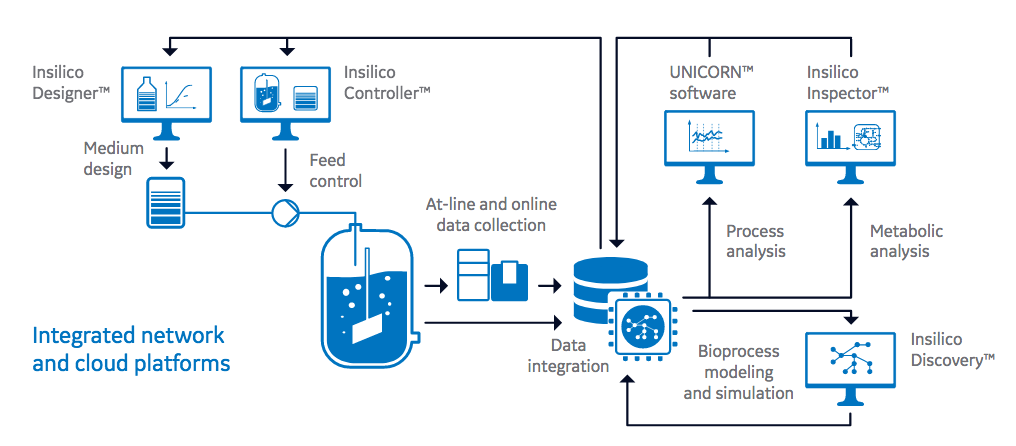
bioprocess data are data management systems that integrate online bioprocess data and at-line data (such as cell
number and viability, metabolites, and product titer and quality). Intelligent software solutions check and analyze
these data sets on the basis of metabolic cell and process models to either optimize the conditions for a specific
clone and product, or to continuously improve the production platform as a whole. In the future, predictive metabolic
modeling will allow for online preemptive process optimization and control based on reduced data sets.
These models are then used to create predictive simulations, which when successfully implemented, can speed process development and reduce the number of required “wet experiments”. These powerful software applications are providing more accurate models and are reducing the amount of time needed to generate the models. Figure 2 provides an example of predictive modeling for simulation of an antibody production process in CHO cells.
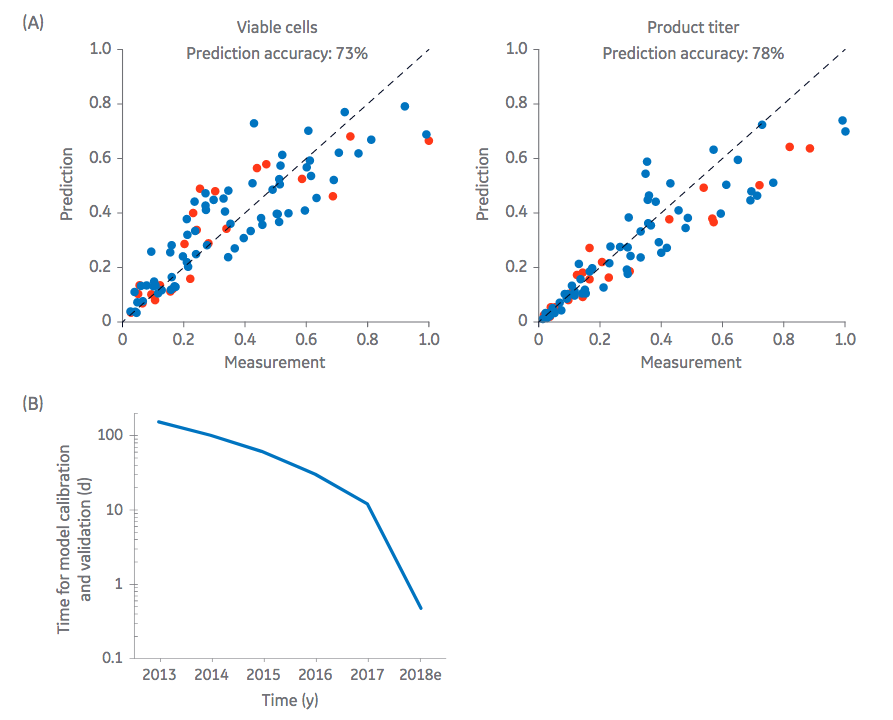
(CHO) cells was used for simulation of an antibody bioproduction process. Comparison of predicted and measured
parameters for a training data set (blue dots) and validation data set (red dots) revealed prediction accuracies of 73%
and 78% for cell density and product titer, respectively. (B) Improved automated workflows and machine learning
algorithms lead to a dramatic decrease in the times required for metabolic model calibration and validation, allowing
for at-line predictive computing.
I was fortunate to be able to speak to one of the authors, William Whitford, GE Healthcare, about digital biomanufacturing and its incorporation into current processes. Below is a transcript of our interview.
One area that is frequently talked about is how to efficiently collect process data and then how to mine the large amounts of collected data for information that will further process development. Can you talk about how digital manufacturing is impacting this area?
The collection, organization and application of large amounts of data is a major element of the 4.0 initiative. Just one enabling technology here is termed “big data analytics”. Generally, this refers to the use of very large amounts of structured and unstructured data from many sources in statistical and mathematical algorithms. In bioprocessing, this data can come from such activities as published cellular metabolic pathways, flux and gene regulation; at-line or even on-line data from PD and manufacturing; early PD data from outside of the final design space; historic process data from validated production runs; process data from other sites running the same platform; or even data from disparate but related platforms. The term also includes a number of technologies enabling, e.g., the creation of predictive mechanistic and machine-learning based models from this type of data.
Could you provide a specific example of the implementation of digital biomanufacturing in process development?
In our product development laboratories, GE Healthcare has used statistical inference and mathematical modeling in developing upstream perfusion processes. They have provided value in establishing how such variables as base media, feed mixtures, cell density and cell-specific perfusion rates influence such outcomes as cell viability, culture duration, process robustness, productivity and product quality. Successful correlation of small-scale pseudo-perfusion data to manufacturing-scale ATF and TTF near-steady state systems validate this approach. In general, predictive mechanistic models have proven their applicability to optimize media, feeding regimes, and process parameters in fed-batch, batch, and perfusion mode.
In the article you talk about the development of intelligent software. In your research did you find that companies are developing this internally to meet their specific needs or are service providers developing the software?
In biomanufacturing this intelligent software could support the ability to spot patterns in production data, compare them to a current process and use this knowledge to accomplish such tasks as to control, optimize and predict outcomes or to schedule subsequent steps. It could integrate with an ERP to help find patterns of external suppliers leading to more robust performance, streamlined process schedules, lower materials inventory and better materials quality. Many of the larger biopharmaceutical manufacturers are now working on such programs to support their particular platforms. Software suppliers have commercialized more adaptable applications featuring various levels of capability, with such names as BioAnalystTM, Augmented Intelligence®, Vertica Analytics, Mindspere, and UnicornTM and InsilicoTM Biotechnology.
To what degree do you think predictive modeling can replace wet experiments?
For bioproduction the answer is a bit more nuanced than small molecule chemistry. First of all, there are orders of magnitude more reactions going on in a bioreactor than in most chemical reactors. Then, there are significantly different types of models we may wish to apply, depending upon what prosses the experiments are testing, and how accurate the answers must be in order to be valuable. We are successfully modeling some process development experiments, in some conditions, right now. For example, the development of a design space is a model of sorts. It predicts the accaptable performance of your culture within the multi-dimensional space you’ve established. But, one big challenge to investing in a powerful and accurate model of a cell culture is that each platform and clone behaves a bit differently in response to process conditions. As new sets of data are generated to build a good model for one clone, it will only be accurate in some ways, and to some degree, when applied to another clone. Thus, while it may seem difficult (from a theoretical point of view) to develop one model that fits all purposes and conditions, there are nowadays robust and automated solutions that enable creation of predictive models from rather limited sets of standard bioprocess data. Moreover, empowered by artificial intelligence, such learning models have even been shown to accommodate different clones, products, and process modes.
Could you explain more about the digital twin concept and how/when it might be implemented?
Well, we’ve spoken a bit about process modeling and the GE Bioreactor Digital Twin is a type of model. It’s actually a virtual representation of the overall bioprocess occurring in the bioreactor. Bioreactor Digital Twins are mixed models of the interplay of the biology and physics of the overall cell culture process using the power of machine learning. This means these digital twins can self-learn and are not simply a static model providing a very specific process under very specific conditions. In fact, each production run will have its own twin created. This twin will then (eventually) support such activities as process monitoring and deviation detection as well as feeding, harvest, product quality and titer predictions. During process development the twin can also aid in optimizing DoE, scaling of the process, clone selection, media optimization and feeding optimization.
Please see related articles: