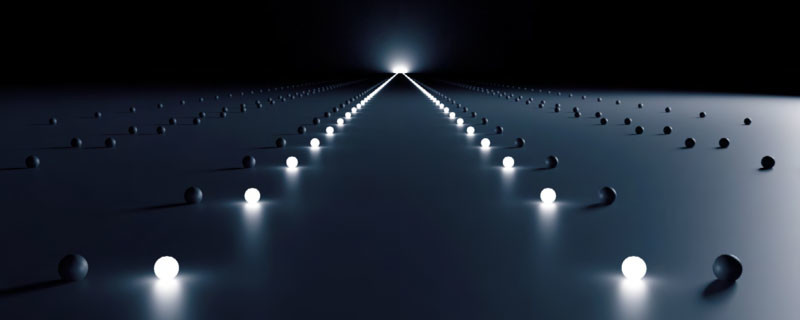
Proteogenomic Strategies to accelerate Drug Discovery and Increase Success
There are countless diseases that are in desperate need of new, more effective therapeutics to address unmet needs or to improve current standards of care. Addressing these needs is no easy task considering the long timeline to approve new drugs coupled with how few drugs successfully make it through clinical trials in the first place.
The challenges of moving a therapeutic candidate through clinical trials was highlighted in the report Biopharmaceutical Research & Development: The Process Behind New Medicines, published by PhRMA. In the report authors’ state that the process for researching and developing new medicines is growing in difficulty and length with the overall probability of clinical success estimated to be less than 12%.
There are many reasons why therapeutics fail in clinical studies. However, the critical first step in the drug discovery and development process is the identification of a disease-causative target (usually a protein) against which a therapeutic agent can be produced. It is essential that the critical selection of drug target is made correctly using the best and most robust data possible, in order to avoid costly failures much later in the development process.
Current Therapeutic Pathway to Approval
To continue the great advancements by the pharmaceutical industry in helping patients, it needs to reduce the timeline for finding effective drug targets while also improving drug target selection to ensure candidates have the highest probability of success.
Using Proteogenomics to Improve Drug Discovery Timelines and Success Rates
New technologies and methods are now being implemented that can make such improvements to the drug discovery process. One such method is to combine large-scale genomics and proteomics (“proteogenomics”), which when combined with phenotypic/clinical data, can be used to identify proteins that are causal in a disease. A proteogenomic approach can also provide a wealth of other data related to disease mechanisms and drug responses that are invaluable for well-informed development of therapeutics. This type of data may be used for example to help predict which subgroup of the patient population will respond to a drug, or to provide surrogate markers for efficacy or safety. Clinical trial success rates can also be increased by stratifying the trial cohort and including only those patients that are likely to respond to the drug. By selecting the right drug target and pairing the resulting candidate drug with the correct patient population, researchers ensure the highest probability of success while preventing the administration of the drug to patient groups that may negatively respond to the drug.
Proteogenomics with Mendelian Randomization to establish disease causality
As discussed previously, establishing causality is very important to drug target identification. Observational studies of protein levels alone cannot establish causality, as any change observed in a specific protein could either be driving the disease under study, or merely reflect an outcome of that disease. In order to move a protein target into drug discovery and development, causality is a prerequisite. Causality can be demonstrated by using proteogenomics to link gene variants to protein expression levels. These types of statistically significant associations are called protein Quantative Trait Loci (pQTLs).
The associations of pQTLs with phenotypic outcomes can be tested in a Mendelian Randomization analysis. Mendelian randomization analysis is a method that uses genetic variants to assess causality of a risk factor on disease. In cases where the genetic variant is located close to the gene encoding the protein of interest (“cis-pQTLs”), this strongly infers causality for the protein in the disease or biological process under investigation. This is because while protein expression could change as a result of disease, the genetic variant cannot. The unique power of this approach has led to its increasing adoption in recent studies, and has also resulted in a major international consortium, SCALLOP.
SCALLOP is a collaborative framework for the discovery and utilization of genetic associations with proteins using the Olink Proteomics platform. The aim of the SCALLOP consortium is to identify novel molecular connections and protein biomarkers that are causal in diseases, and to date, 29 PIs from 24 research institutions have joined the effort, which now comprises summary level data on single nucleotide polymorphism (SNP) to protein level associations from almost 65,000 patients or controls. A landmark publication from the consortium looking at pQTLs for 90 cardiovascular proteins in 30,000 individuals was recently published in Nature Metabolism1.
Next Generation Proteomics Platform Supports Proteogenomics and Multiomics Approaches
One challenge to the implementation of proteogenomics approaches has been the ability to efficiently conduct large-scale protein analysis. Olink has provided a solution to these protein analysis challenges with their proximity extension assay (PEA) technology. PEA technology solves many of the limitations of traditional proteomics, enabling the study of many proteins simultaneously with single-plex quality, high specificity, and sensitivity. It also covers a broad dynamic range, with minimal sample volume required for analysis and perhaps most importantly for the large-scale analysis required for pharma applications, the studies can now be run with very high throughput.
PEA technology combines an antibody-based immunoassay with a digital readout using either quantitative real-time PCR (qPCR) or Next Generation Sequencing (NGS). The unique attributes of this technology support a scalable, multiplex, and highly specific approach where the concentration of up to almost 1500 protein biomarkers can be quantified simultaneously. PEA is a dual-recognition immunoassay, where two matched antibodies labeled with unique DNA oligonucleotides simultaneously bind to a target protein in solution. This brings the two antibodies into proximity, allowing their DNA oligonucleotides to hybridize, which serves as a template for a DNA polymerase-dependent extension step. The resulting double-stranded DNA “barcode” is unique to each specific antigen and quantitatively proportional to the concentration of the target protein in the original sample. Hybridization and extension are immediately followed by PCR amplification. This provides a highly scalable technique with exceptional specificity. Because the PEA assay requires extremely low sample volumes (less than 3 µL to measure ~1500 proteins) it is invaluable in applications where the volume of precious samples is limited, e.g., pediatric samples, micro-biopsies, exosomes, etc.
Olink platform solutions address a wide range of applications and scales. Their solutions include:
- Explore
- Explore 1536 measures almost 1500 proteins in less than 3 microliters of sample and can provide up to 1.35 M protein measurements per week/system.
- The Explore 1536 library is also available as four 384-plex panels focused on key disease areas (Cardiometabolic, Inflammation, Neurology and Oncology).
- Target
- Target 96 provides fifteen carefully designed panels to choose from, all built for specific disease areas or key biology processes.
- Target 48 currently offers a 48-plex cytokine panel with absolute quantification for careful monitoring of the immune system.
- Focus is a custom-designed panel for specific needs. Simultaneously measure up to 21 proteins with relative or absolute quantification for verification and implementation of a protein signature discovered by Olink.
Summary
The pharmaceutical industry has been plagued by drug discovery processes that are both lengthy and prone to high failure rates. The use of proteogenomics technologies and methods seeks to resolve this. By using proteomics with other omics such as genomics, researchers can make critical decisions early about whether a protein target is worth pursuing, by establishing protein causality in the disease of interest. Extending similar approaches across the drug discovery and development process can provide invaluable insights into disease biology and drug action, helping to ensure more rapid production of safe, effective drugs and ensuring their use for the right patient population. As a result, companies save money and time by the early identification of targets that are unlikely to be successful, thereby prioritizing focus and resources on candidates with the highest likelihood of success.
To learn more, please see:
Related articles:
- Next Generation Proteomics is the Missing Piece in Completing the Precision Medicine Puzzle
- How a Next Generation Proteomics Platform is Accelerating the Fight Against COVID-19